* Watch more IBM interview videos *
I recently had the privilege of sitting down with one of our key partners – Srinivas Tummalapenta, the CTO for IBM’s Security Services – to discuss the evolving landscape of AI security. In a world where AI has permeated all sectors, security concerns have emerged as a primary focus. The conversation spanned everything from the shared responsibility model for AI to the emerging need for governance, the importance of AI security posture management, and the industry’s future direction. Following is a summary of the discussion, along with a couple video clips.
AI’s Dual Role in Security
Srini opened the conversation by detailing IBM’s proactive stance in the security landscape. As AI continues to drive productivity and innovation, it also brings unique security challenges. On one hand, AI can be harnessed to automate threat detection and response, bolstering an organization’s defensive capabilities. But equally pressing is the need to secure AI applications themselves. Srini emphasized that IBM is heavily invested in both aspects: using AI to improve security and, crucially, ensuring that AI systems are secure from external threats.
The use of generative AI (GenAI) has amplified these concerns, as many organizations are integrating AI into applications like SAP, Salesforce, and even bespoke AI models. To help clients navigate this landscape, IBM emphasizes a dual approach: using AI effectively while embedding security and compliance into every stage of AI deployment.
Is AI Outpacing Security?
A core theme of the discussion was whether AI’s rapid adoption has outstripped security measures. Srini likened the situation to the cloud computing revolution, where initial enthusiasm often preceded robust security implementations. IBM’s recent study, conducted through the Institute of Business Value, highlighted a concerning gap: although 70% of surveyed executives recognized the criticality of security in AI adoption, only 24% had allocated budgets specifically for AI security projects. This gap between awareness and action is an opportunity for security vendors and consultants to step in and emphasize the risks of underestimating AI security needs.
The lesson from cloud adoption is clear: enterprises must build security measures into their AI strategies from the outset, rather than playing catch-up once vulnerabilities have been exposed.
The AI Shared Responsibility Model
One of the most illuminating aspects of the interview was the discussion around the AI Shared Responsibility Model, which Srini compared to the familiar model used in cloud security. He outlined three common AI consumption patterns:
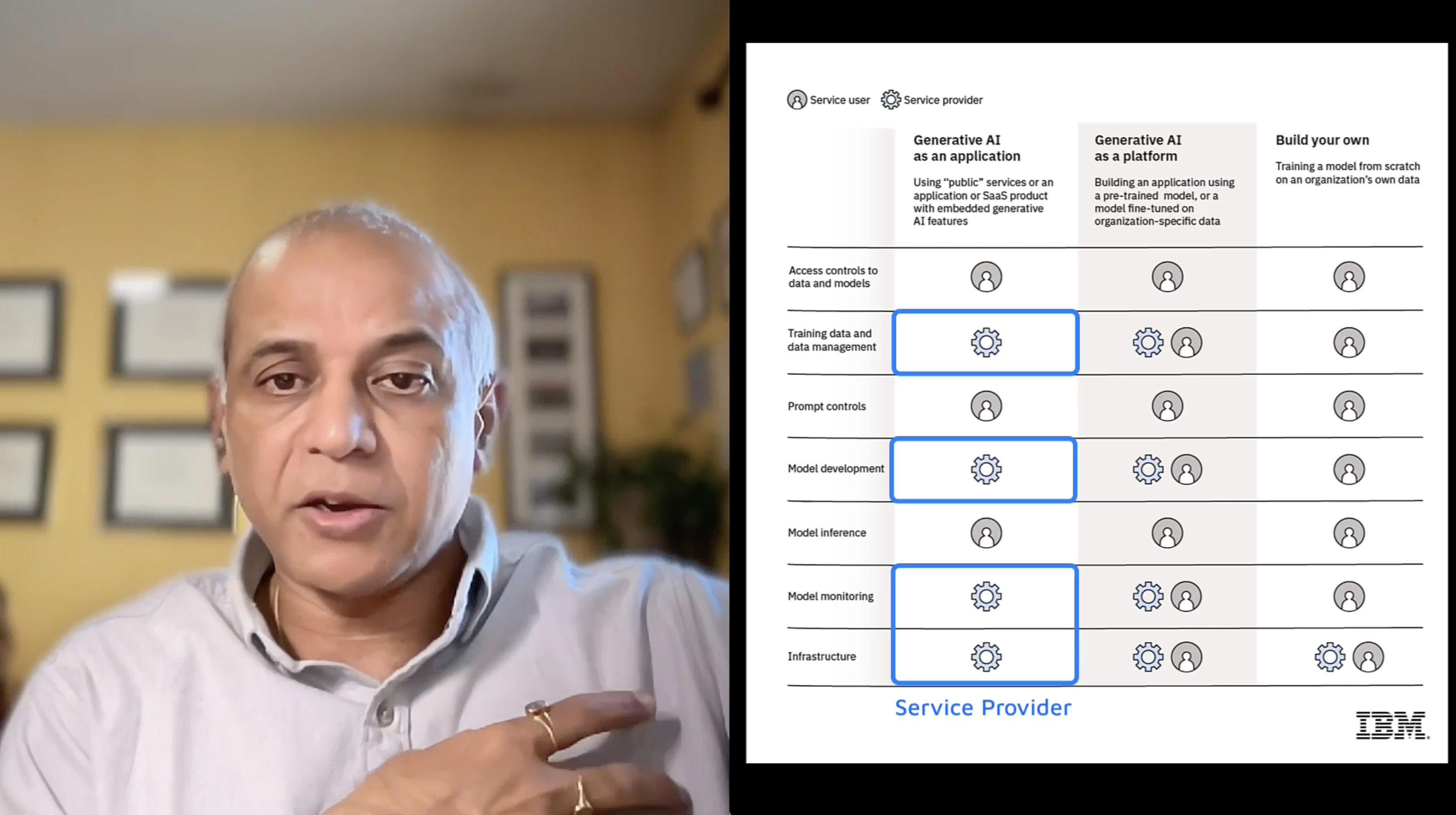
- AI as a Service (AIaaS): This involves using AI models offered by third-party providers, such as ChatGPT or Salesforce. Here, the service provider handles everything up to the model’s inference point. However, the responsibility of securing the data inputs, authentication mechanisms, and API connections falls on the client.
- Hybrid AI Models: Companies use cloud-based infrastructure to fine-tune or customize AI models with their data. In this case, security responsibilities are split. The provider manages infrastructure and base model security, while the client must secure their data and application-specific AI implementations.
- Building AI Models from Scratch: Organizations that build models from the ground up, whether on-premises or in the cloud, bear the full security responsibility. This includes everything from infrastructure to data protection, model governance, and application security.
Srini stressed the importance of assessing which model an organization uses and tailoring security measures accordingly. IBM provides a comprehensive framework to guide clients through this assessment.
Addressing Limited Visibility for CISOs
Another major challenge is the limited visibility that Chief Information Security Officers (CISOs) have into AI projects. Much like the early days of shadow IT, there is now “shadow AI.” Security teams may not be aware of all AI initiatives within an organization, especially as teams experiment with new technologies. He advocated for automated discovery processes to build a complete inventory of AI assets, including both sanctioned and unsanctioned projects. This inventory is the foundation for effective governance and policy enforcement, allowing security teams to manage risks proactively.
Mitigating Model Risks and Malware Threats
The conversation also touched on the potential dangers of models from open-source repositories like Hugging Face. With over a million models available, malware and security vulnerabilities are inevitable concerns. IBM’s approach starts with robust discovery mechanisms to identify all models in use. Once identified, these models undergo thorough security scans, much like traditional software vulnerability assessments.
IBM’s concept of MLSecOps is emerging as a best practice. This approach integrates model and data security into the development pipeline. It involves scanning data for sensitive or prohibited information and validating models for malware and vulnerabilities. The process ensures that AI models are safe before they are put into production, creating a seamless integration with existing DevSecOps practices.
The Role of AI Security Posture Management
Also discussed was the emerging field of AI Security Posture Management (AI SPM). Srini clarified that AI SPM builds upon traditional cloud and application security posture management. However, it specifically addresses AI-related assets, like models and training datasets, as well as the unique security configurations required for these elements.
A holistic approach is essential. While cloud security focuses on infrastructure, AI SPM ensures that models and data remain secure throughout their lifecycle. This includes preventing data poisoning attacks and ensuring that models are not tampered with, both of which could have catastrophic consequences if left unchecked.

The Unlearning Challenge and Emerging Regulations
One intriguing issue raised was the concept of “unlearning.” Current AI systems lack a straightforward way to unlearn information if, for example, they have inadvertently trained on sensitive or unauthorized data. Regulations like the European Union’s GDPR make unlearning a critical requirement, yet practical solutions remain elusive. IBM is researching ways to address this, but it’s a complex challenge that highlights the evolving regulatory landscape.
Global AI regulations are becoming increasingly ubiquitous and complex. With countries and even individual states drafting unique laws, organizations must stay vigilant and adaptive. Starting with frameworks like the EU AI Act or NIST’s Risk Management Framework can provide a solid foundation. Yet, as regulatory scrutiny increases, enterprises must continuously refine their policies.
A Roadmap for AI Security
To wrap up, Srini shared IBM’s “subway map” analogy for guiding organizations on their AI security journey. This roadmap outlines the various stages of AI development—strategy, design, build, and run—and the corresponding security measures needed at each phase. The goal is to help organizations take a structured approach, reduce anxiety, and prepare for the evolving AI landscape.
Ultimately, the key to AI security lies in collaboration, continuous learning, and proactive governance. As AI becomes more ingrained in business operations, the conversation around securing it will only grow more critical.
* Watch more IBM interview videos *